Batch Processing
As a data enthusiast and someone who has spent years navigating the intricate world of data management, I’ve had my fair share of experiences with batch processing. One memorable instance was during a large-scale data migration project for a retail client, where we had to transfer millions of customer records from an outdated system to a modern database. The challenge was daunting, but the implementation of batch processing turned what could have been a chaotic endeavor into a streamlined operation.
Imagine a bustling retail store during the holiday season. Customers are flocking in, and the registers are ringing. Now, picture the backend systems working tirelessly to process all that data in real-time. But what happens when the store closes? This is where batch processing comes into play, allowing businesses to efficiently handle large volumes of data after hours, ensuring everything is up-to-date for the next day.
A Glimpse into History
The concept of batch processing dates back to the early days of computing, when mainframes were the giants of data handling. Initially, data was processed in large batches due to the limitations of hardware and software. Over the years, as technology evolved, so did the methods of processing data. Today, batch processing remains a cornerstone of data management in various industries, proving its relevance even in an era dominated by real-time processing.
Engaging the Reader
Have you ever wondered how massive amounts of data are transferred seamlessly across systems without causing a ripple in operations? The answer often lies in the efficient use of batch processing.
Understanding Batch Processing in Data Management
Defining the Core Concept
At its essence, batch processing refers to the execution of a series of jobs on a computer without manual intervention. This method is particularly crucial in data-driven environments where the volume of data can overwhelm traditional processing methods. By grouping data into manageable batches, organizations can optimize performance and resource utilization.
Interacting with EDI, RFID, and Integration Technologies
Batch processing interacts seamlessly with technologies like Electronic Data Interchange (EDI) and Radio Frequency Identification (RFID). For instance, EDI allows businesses to exchange documents electronically, and when combined with batch processing, it enables the efficient transfer of large datasets, such as invoices or purchase orders, in one go. Similarly, RFID technology can be used to gather data in bulk, which can then be processed in batches for inventory management.
Practical Use Cases Across Industries
The versatility of batch processing is evident across various sectors:
- Logistics: Shipment tracking and inventory updates are often handled in batches to ensure accuracy and efficiency.
- Finance: Banks utilize batch processing for end-of-day reconciliations, processing thousands of transactions at once.
- Healthcare: Patient records are updated in batches, ensuring that all information is current and accessible.
- Retail: Sales data is aggregated in batches to analyze trends and inform stock replenishment strategies.
Technical Features & Implementation
Key Functionalities and Components
Understanding the core functionalities of batch processing is essential for effective implementation. Key components include:
- Job Scheduling: Automating the execution of batch jobs at specified times.
- Data Transformation: Converting data into a suitable format for processing.
- Error Handling: Mechanisms to manage failures and ensure data integrity.
Integration Methods, APIs, and Protocols
Integrating batch processing into existing systems can be achieved through various methods, including:
- APIs: Application Programming Interfaces allow different software systems to communicate and share data efficiently.
- Protocols: Standardized methods of communication, such as HTTP or FTP, facilitate the transfer of batch data.
Comparing Different Approaches
When it comes to handling data migration, several approaches exist. Below is a comparison chart showcasing various technologies:
- Traditional Batch Processing: Scheduled jobs that run at specific intervals.
- ETL (Extract, Transform, Load): A process that extracts data from one source, transforms it, and loads it into another.
- Real-Time Processing: Continuous input and processing of data, often used in conjunction with batch methods for comprehensive data management.
Challenges & Solutions in Data Transfer
Common Challenges
Implementing batch processing is not without its hurdles. Common challenges include:
- Data Quality: Ensuring the accuracy and consistency of data before processing.
- Scalability: As businesses grow, so do their data needs, requiring scalable batch solutions.
- Integration Complexity: Merging batch processing with existing systems can be intricate and time-consuming.
Best Practices for Seamless Integration
To overcome these challenges, consider the following best practices:
- Conduct thorough testing before deployment to identify potential issues.
- Utilize monitoring tools to track batch job performance and identify bottlenecks.
- Implement robust security measures to protect sensitive data during transfer.
Security Concerns and Compliance Requirements
With the rise of data breaches, security is paramount. Organizations must adhere to compliance requirements such as GDPR or HIPAA when handling sensitive information. This necessitates implementing encryption and secure access controls during batch processing.
Expert Insights & Industry Trends
Expert Opinions
“Batch processing remains a vital tool in the data management arsenal, especially for organizations dealing with large datasets. Its efficiency and scalability are unmatched.” – Data Management Expert
Case Studies
Consider a case study of a major bank that adopted batch processing for their nightly transaction reconciliations. They reported a 30% reduction in processing time and improved accuracy in their financial reports.
Emerging Trends
As technology advances, trends such as cloud computing and machine learning are shaping the future of batch processing. These innovations facilitate faster data processing and enhanced analytical capabilities.
Final Thoughts & Practical Applications
Key Takeaways
In summary, batch processing plays a pivotal role in data transfer, migration, and integration. Its ability to handle large volumes of data efficiently makes it indispensable in today’s data-driven world.
Recommendations
For businesses looking to adopt and optimize batch processing, consider the following recommendations:
- Invest in training for staff to understand the intricacies of batch processing.
- Utilize modern tools and software that support batch processing capabilities.
- Regularly review and update your batch processing strategies to align with industry best practices.
Resources for Better Data Management
Here are some resources and tools that can assist businesses in enhancing their data management:
- Apache Hadoop: A powerful framework for processing large data sets.
- Talend: An open-source data integration tool that supports batch processing.
- Informatica: A comprehensive data management solution for enterprises.
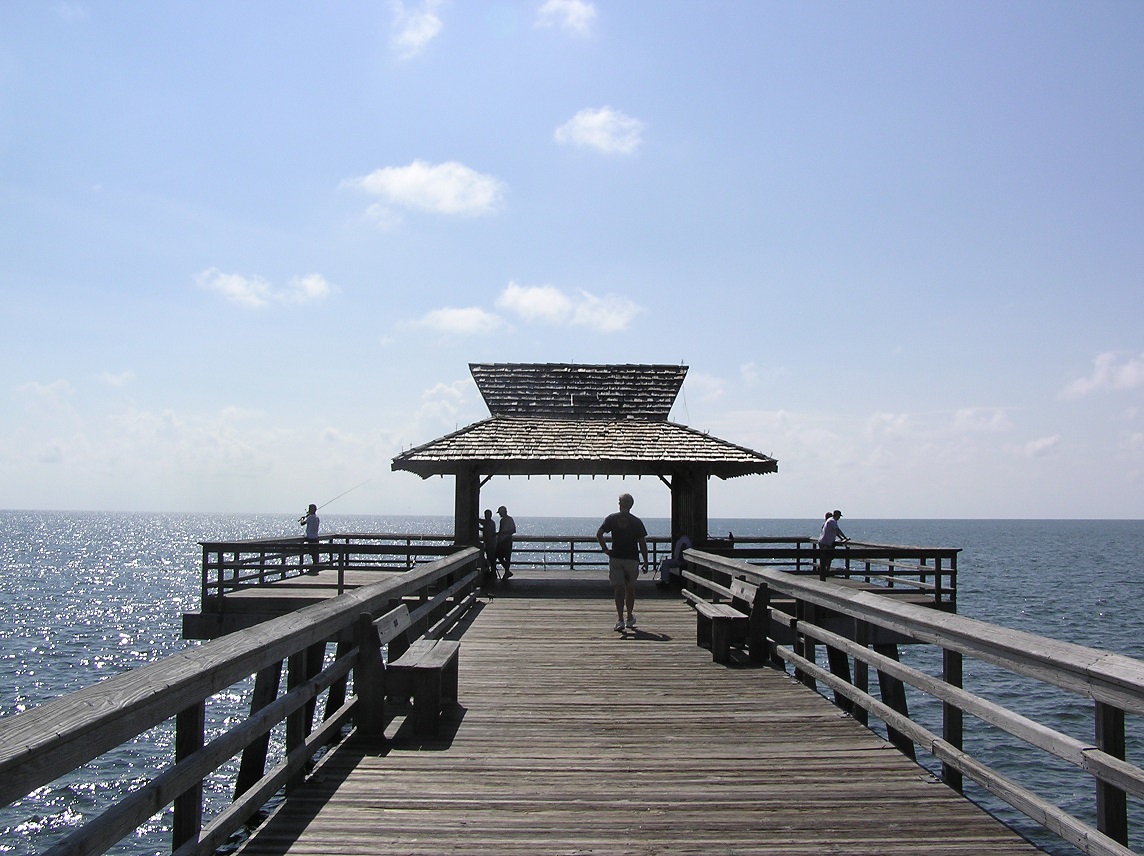
Owner/Author of UCCnet.org. Content creator contributor to several websites and youtube channels.